Banks as Technology Platforms: An Evolution?
The transformation of banks into technology platforms is not merely a trend but rather a necessity rooted in the ever-evolving landscape of the financial industry. As digitalization accelerates, the competition from tech giants such as Amazon, Google, and various fintech companies throws banks into a precarious situation. Essentially, the future of banking hinges on the relationship dynamics between these financial institutions and regulatory bodies.
Historically, banks have thrived in an environment where high-interest spreads allowed them to flourish. The 1960s witnessed bankers basking in the glory of their perceived roles as trustworthy stewards of wealth, adhering to the "3-3-3" rule: a 3% interest margin, three hours of lunch with clients, and a 3 PM golf outing. However, following the 2008 financial crisis, the blanket of safety covering banks began to unravel. With the shift in U.S. Federal Reserve and European Central Bank policies of slashing interest rates to stimulate the economy, the margin slices that banks once enjoyed began to narrow significantly. Ensuing economic downturns and a rise in non-performing loans shredded any semblance of stability, and banks found themselves facing a stark reality.
The emergence of technology and fintech platforms at the start of the 21st century invoked a somewhat dismissive response from banks and financial regulators regarding the potential threats posed by such innovations. Initially, fintech companies seized the opportunity to dominate the payments ecosystem, which quickly transformed into a turf war over loan services. These tech platforms outmaneuvered traditional banks by offering clients what they sought—quicker, more efficient financial services delivered with reduced operational costs and no burdensome legacy infrastructure.
Advertisement
According to McKinsey, by the close of 2019, the cumulative market capitalization of the world’s 200 largest banks ranged between 0.8 to 1.2 relative to their book value, starkly contrasting with the leading technology companies whose price-to-book ratios exceeded 5. Both entities shared similar market capitalizations of about $6 trillion. Today, JPMorgan Chase claims the title of the largest bank by market value, positioned at a humble 14th place globally, while Tech “Big Seven” and Aramco soars beyond the $1 trillion threshold.
Diving deeper, contrast the price-to-earnings ratios: China's Industrial and Commercial Bank stands at a mere 4.5 against JPMorgan's 12.3, while tech giants such as Google and Nvidia boast ratios of 23 and 66, respectively. Historically, banking assets dominated the financial ecosystem. However, stringent capital requirements and tighter regulations have kept traditional banks from swiftly expanding their reach compared to non-bank financial intermediaries—shadow banks that thrived post-2008. As of the end of 2022, bank assets represented 39.7% of global financial assets, overshadowed by NBFIs at 47.2%.
Boston Consulting Group pinpoints the necessity for banks to boldly embrace technology to regain their former market values. The routes to reviving profitability and expanding market share are threefold. Firstly, they can transition from traditional interest products towards non-interest revenue streams, such as wealth management and private banking. Secondly, banks can venture into foreign markets along with currencies, bonds, and derivatives. Lastly, leveraging technology to drive down operational costs while enhancing customer service is of paramount importance.
The sobering statistics indicate that U.S. banks have experienced an average annual decrease of 0.3% in productivity since 2010, contrasting sharply with rising productivity across other industries. Nonetheless, 2023 witnessed banks pouring about $650 billion into technology—an investment that parallels the GDP of Belgium. Struggling to pivot outdated mindsets toward embracing online service paradigms, banks have shown ineptitude in competitive online environments. The ingrained risk-averse behaviors of bankers coupled with regulatory caution create an atmosphere stifling technological innovation.
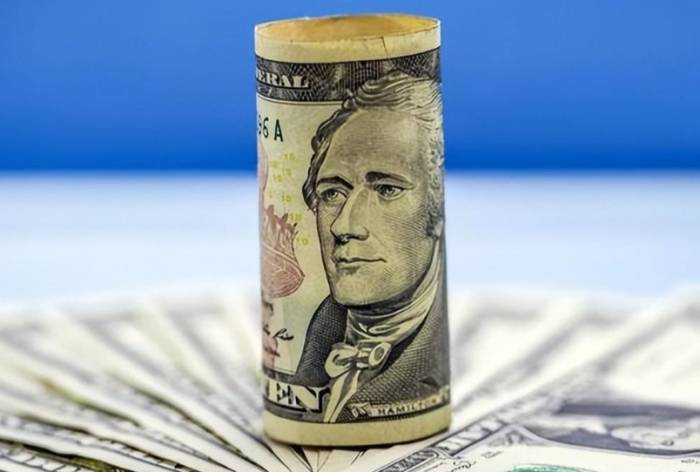
The notion of a regulatory "sandbox," designed to protect banks while they experiment with technology, likens them to children with training wheels, resulting in dependence on approvals rather than engaging fully with the competitive tech landscape. Notably, former Federal Reserve Chair Paul Volcker succinctly summed up the technological stagnation in banking by stating, “The ATM has been the only meaningful innovation in banking over the last 20 years.”
Currently, artificial intelligence (AI) marks a pivotal moment in transforming the financial realm. Rapid advancements in online and offline banking service integration are reshaping expectations, with intelligent chatbots alleviating frustrations historically tied to traditional call centers. Gone are the days when accessing simple information was fraught with menus and long waiting times.
Nevertheless, banks akin to Credit Suisse or Silicon Valley Bank struggling with systemic failures find it hard to envision a future where they transform into tech product providers with multiplied valuations. The modern landscape's demands necessitate leaders who think and operate like innovators rather than cautious bureaucrats draped in convoluted financial jargon. Today’s bank CEOs often emerge from CFO backgrounds, rather than from technological prowess.
Fundamentally, banks have confined their focus to the application of technology within finance—pursuing progressively slimmer margins in payments and traditional offerings—rather than embracing the broader concept of 'fintech' as transforming the very essence of financial transactions. Tech platforms foster narratives of transformation and profitability, juxtaposed against banks clinging to defensive approaches characterized by outdated technologies.
We see parallels in the automotive industry, where innovative electric vehicle producers like Tesla have valuations soaring with a P/E ratio of 60, versus Toyota's modest 7. This phenomenon illustrates a failure among traditional company leaders to grasp the competitive threats posed by evolving technologies, thereby resulting in an inadequately responsive narrative to what could be a compelling company story.
Boston Consulting Group projects that banks' digital transformation encapsulates a staggering opportunity amounting to $7 trillion. However, this transformation occurs against a backdrop of heightened capital demands and regulatory constraints, coupled with elevated risks. Global financial infrastructures, including the SWIFT payment system and other clearing mechanisms, require updates while grappling with geopolitical strains. Expectations from governments and consumers for banks to play leading roles in climate change responses and green transitions add additional layers of complexity and cost allocation.
Ultimately, whether banks can pivot into bona fide technology platforms while navigating collaborative relations with regulators is a question central to the industry. Simultaneously, the encroachment of tech giants looms ever larger, promising to capture an increasing share of market territory previously held by traditional banking institutions.
Post Comment